AI development relies upon on us the usage of much less information, now no longer greater
In the information technological know-how network, we’re witnessing the beginnings of an infodemic
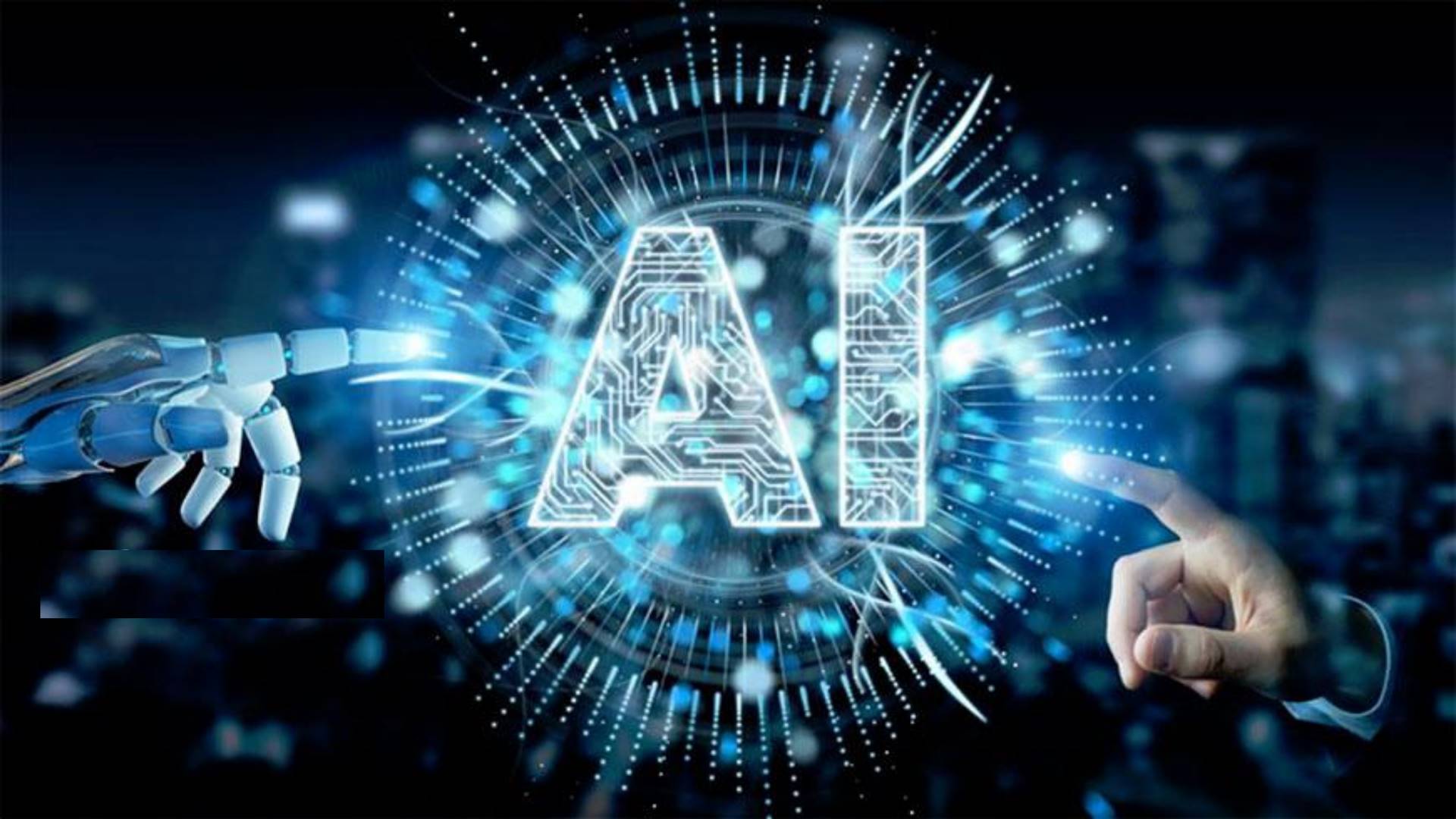
— in which greater information turns into a legal responsibility as opposed to an asset. We’re constantly shifting toward ever-greater information-hungry and greater computationally pricey today’s AI fashions. And this is going to bring about a few unfavorable and possibly counter-intuitive side-effects (I’ll get to the ones shortly).
To keep away from critical downsides, the information technological know-how network has to begin running with a few self-imposed constraints: mainly, greater restricted information and computing assets.
A minimal-information exercise will allow numerous AI-pushed industries — inclusive of cyber safety, that’s my personal region of focus — to emerge as greater green, accessible, independent, and disruptive.
When information turns into a curse as opposed to a blessing
Before we pass any further, permit me to provide an explanation for the trouble with our reliance on an increasing number of information-hungry AI algorithms. In simplistic phrases, AI-powered fashions are “mastering” without being explicitly programmed to do so, thru a tribulation and mistakes procedure that is based on a collected slate of samples. The greater information factors you’ve got – despite the fact that lots of them appear indistinguishable to the bare eye, the greater correct and strong AI-powered fashions you have to get, in theory.
In seek of better accuracy and coffee false-fine rates, industries like cyber safety — which changed into as soon as constructive approximately it’s capacity to leverage the unheard-of quantity of information that accompanied from organization virtual transformation — are actually encountering an entirely new set of challenges:
1. AI has a compute addiction. The developing worry is that new improvements in experimental AI studies, which often require ambitious datasets supported with the aid of using the right compute infrastructure, is probably stemmed because of computing and reminiscence constraints, now no longer to say the monetary and environmental costs of better compute needs.
While we may also attain numerous greater AI milestones with this information-heavy approach, through the years, we’ll see development slow. The information technological know-how network’s tendency to the purpose for information-“insatiable” and compute-draining today’s fashions in sure domains (e.g. the NLP area and its dominant massive-scale language fashions) have to function as a caution sign.
OpenAI analyses endorse that the information technological know-how network is greater green at accomplishing desires which have already been received however demonstrate that it calls for greater compute, with the aid of using some orders of magnitude, to attain new dramatic AI achievements. MIT researchers estimated that “3 years of algorithmic development is equal to a ten instances boom in computing power.” Furthermore, developing an ok AI version so one can resist concept-drifts through the years and overcome “underspecification” normally calls for more than one round of schooling and tuning, because of this that even greater compute assets.
If pushing the AI envelope method eating even greater specialized assets at extra costs, then, yes, the main tech giants will preserve paying the fee to live withinside the lead, however maximum educational establishments might locate it hard to participate in this “excessive risk – excessive reward” competition. These establishments will maximum probably both embody resource-green technology or pursue adjoining fields of studies. The tremendous compute barrier would possibly have an unwarranted cooling impact on educational researchers themselves, who would possibly pick out to self-restraint or absolutely chorus from pursuing progressive AI-powered improvements.
2. Big information can suggest greater spurious noise. Even in case you count on you’ve got well described and designed an AI version’s goal and structure and which you have gleaned, curated, and thoroughly organized sufficient applicable information, you don’t have any warranty the version will yield useful and actionable outcomes. During the schooling procedure, as extra information factors are consumed, the version would possibly nevertheless discover deceptive spurious correlations among distinct variables. These variables are probably related in what appears to be a statistically tremendous manner, however aren’t causally associated and so don’t function beneficial signs for prediction purposes.
I see this in the cyber safety field: The enterprise feels pressured to take as many capabilities as viable into account, in the desire of producing higher detection and discovery mechanisms, safety baselines, and authentication processes, however, spurious correlations can overshadow the hidden correlations that really matter.
3. We’re nevertheless the handiest making linear development. The reality that massive-scale information-hungry fashions carry out thoroughly beneath neath particular occasions, with the aid of using mimicking human-generated content material or surpassing a few human detection and popularity skills, is probably deceptive. It would possibly impede information practitioners from figuring out that a number of the present-day efforts in applicative AI studies are handiest extending current AI-primarily based totally skills in a linear development as opposed to generating actual leapfrog improvements — in the manner companies stable their structures and networks, for example.
Unsupervised deep mastering fashions consumed massive datasets have yielded awesome outcomes over the years — in particular thru switch mastering and generative antagonistic networks (GANs). But even in mild of development in neuro-symbolic AI studies, AI-powered fashions are nevertheless some distance from demonstrating human-like intuition, imagination, pinnacle-down reasoning, or synthetic well-known intelligence (AGI) that would be implemented widely and efficiently on basically distinct problems — including varying, unscripted, and evolving safety duties whilst going through dynamic and complex adversaries.
4. Privacy issues are expanding. Last however now no longer least, collecting, storing, and the usage of full-size volumes of information (inclusive of user-generated information) — that’s in particular legitimate for cyber safety applications — increases a plethora of privateness, prison, and regulatory issues and considerations. Arguments that cyber safety-associated information factors don’t bring or represent for my part identifiable information (PII) are being refuted those days, because the robust binding among private identities and virtual attributes are extending the prison definition PII to include, for example, even an IP address.
Meet Robby Megabyte, Bosnia and Herzegovina’s first robotic rock musician
How I discovered to prevent trauma and revel in information scarcity
In order to conquer those challenges, mainly in my region, cyber safety, we’ve to, first and foremost, align expectations.
The sudden emergence of Covid-19 has underscored the problem of AI fashions to efficiently adapt to unseen, and possibly unforeseeable, occasions and edge-cases (including an international transition to far off paintings), in particular in our on-line world in which many datasets are clearly anomalous or characterized with the aid of using an excessive variance. The pandemic handiest underscored the significance of certainly and exactly articulating a version’s goal and thoroughly getting ready its schooling information. These duties are normally as essential and labor-intensive as collecting extra samples or maybe deciding on and honing the version’s structure.
These days, the cyber safety enterprise is needed to undergo but any other recalibration section because it involves phrases with its lack of ability to deal with the “information overdose,” or infodemic, that has been plaguing the cyber realm. The following techniques can function as guiding ideas to boost up this recalibration procedure, and they’re legitimate for different regions of AI, too, now no longer simply cyber safety:
Algorithmic efficacy as pinnacle priority. Taking inventory of the plateauing Moore’s law, businesses and AI researchers are running to ramp-up algorithmic efficacy with the aid of using checking out modern techniques and technology, a number of that are nevertheless in a nascent degree of deployment. These techniques, which are presently relevant handiest to particular duties, variety from the utility of Switch Transformers, to the refinement of Few Shots, One-Shot, and Less-Than-One-Shot Learning techniques.
Human augmentation-first approach. By proscribing AI fashions to the handiest increase the safety professional’s workflows and permitting human and synthetic intelligence to paintings in tandem, those fashions can be implemented to very narrow, well-described safety applications, which with the aid of using their nature require much less schooling information. These AI guardrails can be manifested in phrases of human intervention or with the aid of using incorporating rule-primarily based totally algorithms that hard-code human judgment. It isn’t an accident that a developing wide variety of safety providers choose imparting AI-pushed answers that handiest increase the human-in-the-loop, rather than changing human judgment altogether.
Regulators can also appearance favorably in this approach because they search for human accountability, oversight, and fail-secure mechanisms, in particular on the subject of automated, complex, and “black box” processes. Some providers are looking for a center floor with the aid of using introducing lively mastering or reinforcement mastering methodologies, which leverage human enter and knowledge to complement the underlining fashions themselves. In parallel, researchers are running on improving and refining human-system interplay with the aid of using coaching AI fashions while deferring a choice to human experts.
Leveraging hardware improvements. It’s now no longer but clean whether or not dedicated, rather optimized chip architectures and processors along with new programming techniques and frameworks, or maybe absolutely distinct automatic structures, might be capable of accommodating the ever-developing AI computation demand. Tailor-made for AI applications, a number of those new technological foundations that intently bind and align specialized hardware and software, are greater successful than ever of appearing unattainable volumes of parallel computations, matrix multiplications, and graph processing.
Additionally, purpose-constructed cloud times for AI computation, federated mastering schemes, and frontier technology (neuromorphic chips, quantum computing, etc.) can also additionally play a key function in this effort. In any case, those improvements on my own aren’t probably to reduce the want for algorithmic optimization that would possibly “outpace profits from hardware efficiency.” Still, they may show to be critical, as the continuing semiconductor struggle for AI dominance has but to supply a clean winner.
Hitting the Books: AI medical doctors and the risks tiered hospital treatment
The deserves of information discipline
Up to now, traditional awareness in information technological know-how has normally dictated that on the subject of the information, the greater you’ve got, the higher. But we’re now starting to see that the downsides of information-hungry AI fashions would possibly, through the years, outweigh their undisputed advantages.
Enterprises, cyber safety providers, and different information practitioners have more than one incentive to be greater disciplined in the manner they collect, store, and eat information. As I’ve illustrated here, one incentive that has to be the pinnacle of thoughts is the capacity to raise the accuracy and sensitivity of AI fashions whilst assuaging privateness issues. Organizations that embody this approach, which is based on information dearth as opposed to information abundance, and workout self-restraint, can be higher geared up to pressure greater actionable and cost-powerful AI-pushed innovation over the lengthy haul.
Eyal Balicer is Senior Vice President for Global Cyber Partnership and Product Innovation at Citi.
Venurebeat / TechConflict.Com